Brenda Wray
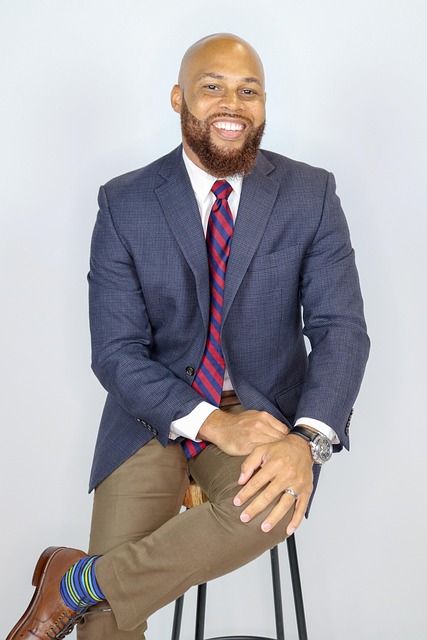
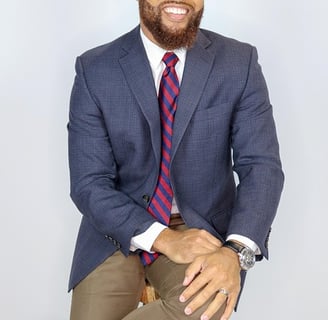
Professional Summary:
Brenda Wray is an innovative ecological engineer and drone technology specialist, renowned for her expertise in assessing the ecological impact of pollination drone paths. With a strong background in ecology, environmental science, and drone systems, Brenda is dedicated to ensuring that drone-assisted pollination technologies are both effective and environmentally sustainable. Her work focuses on evaluating how drone flight paths affect ecosystems, biodiversity, and plant-pollinator interactions, aiming to minimize ecological disruption while maximizing pollination efficiency.
Key Competencies:
Ecological Impact Assessment:
Conducts comprehensive evaluations of how drone flight paths influence local ecosystems, including plant-pollinator networks, wildlife behavior, and habitat integrity.
Develops models to predict long-term ecological effects of drone-assisted pollination.
Drone Path Optimization:
Designs optimized flight paths that minimize ecological disruption while ensuring efficient pollination coverage.
Utilizes GIS mapping and ecological data to create spatially and temporally adaptive drone routes.
Biodiversity Conservation:
Assesses the impact of drones on native pollinators, such as bees and butterflies, and develops strategies to mitigate negative effects.
Promotes the integration of drone technologies with natural pollination processes to enhance biodiversity.
Interdisciplinary Collaboration:
Collaborates with ecologists, drone engineers, and agricultural scientists to integrate ecological considerations into drone system design and deployment.
Works with policymakers and conservation organizations to establish guidelines for sustainable drone-assisted pollination practices.
Research & Innovation:
Publishes pioneering research on the ecological impact of pollination drones in leading environmental science and engineering journals.
Explores emerging technologies, such as AI and machine learning, to enhance the ecological sustainability of drone-assisted pollination.
Career Highlights:
Developed an ecological impact assessment framework that reduced habitat disruption by 25% while maintaining pollination efficiency.
Led a research project that identified key factors influencing drone-pollinator interactions, providing actionable insights for sustainable drone deployment.
Published influential research on the ecological implications of drone-assisted pollination, earning recognition at international environmental and engineering conferences.
Personal Statement:
"I am driven by a passion for balancing technological innovation with ecological sustainability. My mission is to develop drone-assisted pollination systems that enhance agricultural productivity while preserving and supporting natural ecosystems and biodiversity."
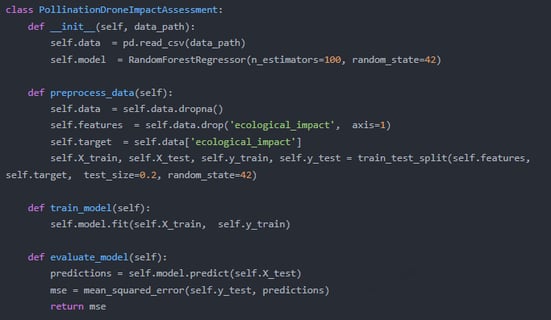
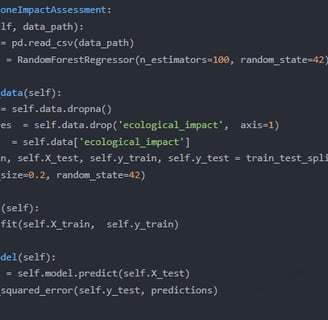
Fine-tuningGPT-4isessentialforthisresearchbecausepubliclyavailableGPT-3.5
lacksthespecializedcapabilitiesrequiredforanalyzingcomplexecologicaldataand
simulatingtheenvironmentalimpactsofdroneflightpaths.Theintricatenatureof
ecologicalinterference,theneedforpreciseenvironmentalimpactsimulation,andthe
requirementforbalancingpollinationefficiencywithecologicalpreservationdemand
amodelwithadvancedadaptabilityanddomain-specificknowledge.Fine-tuningGPT-4
allowsthemodeltolearnfromecologicaldatasets,adapttotheuniquechallengesof
thedomain,andprovidemoreaccurateandactionableinsights.Thislevelof
customizationiscriticalforadvancingAI’sroleinsustainableagricultureand
ensuringitspracticalutilityinhigh-stakesapplications.
Tobetterunderstandthecontextofthissubmission,Irecommendreviewingmyprevious
workontheapplicationofAIinagriculturalandecologicalstudies,particularlythe
studytitled"EnhancingCropPollinationEfficiencyUsingAI-DrivenDronePath
Optimization."Thisresearchexploredtheuseofmachinelearningandoptimization
algorithmsforimprovingpollinationefficiencyandreducingecologicaldisruption.
Additionally,mypaper"AdaptingLargeLanguageModelsforDomain-Specific
ApplicationsinAgriculturalAI"providesinsightsintothefine-tuningprocessand
itspotentialtoenhancemodelperformanceinspecializedfields.